Boon Logic
Boon Logic is a pioneer in the field of artificial intelligence, driven by a mission to enhance human intelligence through cutting-edge technology. Their patented nano-clustering technology has revolutionized unsupervised machine learning, simplifying and accelerating AI development and deployment, particularly in the domains of visual inspection and preventive maintenance.
Test the Amber solution in the Free Trial environment as part of the Smart Equipment Management demo.
AI Anomaly Detection with Amber – a nano clustering technology
The problem
Amber is Boon Logic’s AI-based predictive maintenance solution based on unsupervised machine learning. Amber is self-configuring, runs on commodity servers or in the cloud and trains a unique, high-dimensional model for each asset providing unparalleled predictive accuracy.
Amber has become the first AI-based predictive maintenance tool that can easily be used by plant staff to create accurate and reliable models for predicting equipment failure and identifying its causes.
Amber doesn’t require knowledge of machine learning. Instead, production managers and reliability technicians simply connect Amber to each asset’s sensor telemetry and allow Amber to take it from there and build a unique predictive model for that asset.
Challenges
- AI-based solutions generally require high levels of machine-learning expertise to implement.
- The increasing volume of equipment data requires large cloud-computing architectures.
- To address the sheer number of assets in an environment, one has two options: creating generic, “universal” models or reducing the number of assets they will monitor.
- The complexity and volume of data, along with the computing power needed to process, results in long data engineering and model training cycles.
Solution
- Amber is a machine learning tool purpose-built for reliability and process engineers, who lack AI expertise but are experts in operations.
- Amber is extremely efficient, allowing large volumes of telemetry to be consumed and monitored with minimal compute needed.
- Amber factors in the unique nature of each individual asset, creating distinct models of each of them for optimized accuracy and sensitivity.
- Amber reduces the complexity and time to deploy asset-specific models for your operational environment using a simple 3-step configuration process.
Benefits
- Amber democratizes machine learning for companies lacking data science expertise.
- Amber’s computational efficiency reduces cloud costs commonly associated with the deployment of AI workloads.
- As each asset has its own fingerprint/model tailored to its unique operating environment, Amber delivers better accuracy and sensitivity for earlier detection while greatly reducing false positives.
- By reducing the complexity and time to deploy, Amber allows more models to be built to increase the asset coverage of predictive maintenance.
Amber embedded in Cumulocity
Finding the abnormal is easy once you understand the normal. Even with the most complex equipment, Amber trains from data collected during normal operations instead of relying on failure modes and programmed logic.
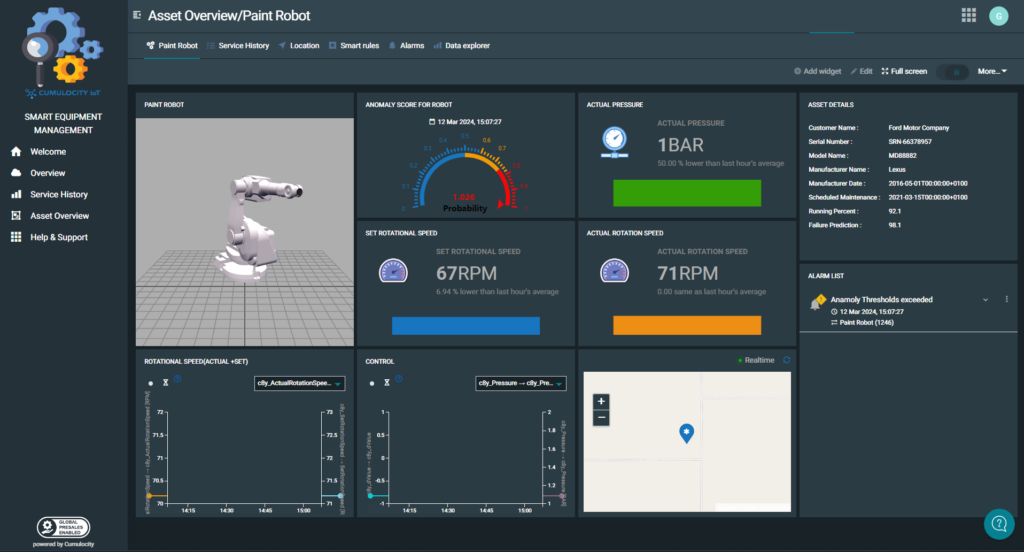
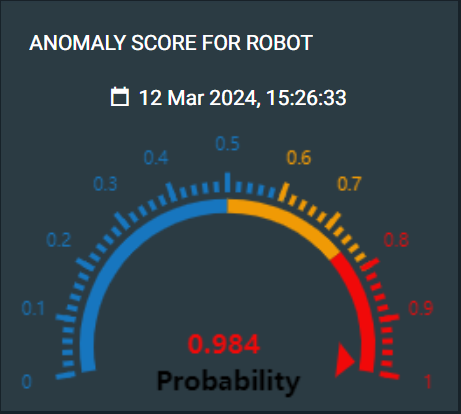
Starting from normal is the only way to have insights you can truly trust. Amber’s Compliance Score is based on an individualized, high-dimensional, unsupervised machine learning model of your asset. The score indicates ongoing asset health and deviations from normal operating behavior. Normal: 51% – 100% Changing: 26% – 50% Critical: 0% – 25%
Alarms can be generate when anomalies are detected. The alarms can be used for service technicians in a connected Smart Field Service Tool.
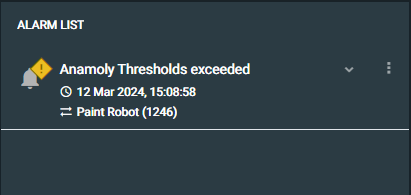